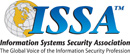
Abstract
Today’s Internet lines are the carriers of electronic business events all over the world. Every Internet-connected business wants to believe that users and customers who conduct business transactions on its website are real and not “artificial users” that emulate human behavior and thus misuse the service in a way that may result in pure revenue loss. This article begins with a short explanation of the main pain points caused by the “artificial user” and the motivation behind the demand to solve them. The main objective of the article is to characterize the technologies that will meet the goals of identifying an “artificial user.” This article ends with an illustration of how these technologies can be useful for the detection and prevention of the artificial user phenomena.
I recently had a few interesting discussions with chief security architects and network security managers of large e-commerce sites in the U.S. They told me that in the past few years they have reached a point at which they are able to identify the sources of attack or the patterns of attack behavior. However, they prefer to allow these attacks to take place – up to a certain level – which is usually set according to the financial risk it may impose on the business. The main reason for this is that after implementing countermeasures, the attacks “respond” almost immediately, thus raising the bar and creating a much more advanced attack technique that is harder to detect and control, which in turn can cause more harm. They also claim that if these cyber criminals are not able to be successful at some level, they will channel their frustration into bringing the site down by generating different types of DoS and DDoS attacks, and in this scenario everybody loses!
Obviously the spectrum of attack types that e-commerce sites need to respond to is vast. However, the main problem they all struggle with today is primarily associated with a phenomenon called the artificial user. These artificial users conduct different types of attacks and, most importantly, try to emulate legitimate user behavior as much as possible. Thus, the main issue businesses are confronted with is how to effectively differentiate between a real user who is trying to perform a legitimate business transaction and an artificial one.
The artificial user also responds very quickly to the defense mechanisms that aim to block it. How so? The designer of the artificial user measures the success versus failure of his attack tool and then decides how to change the software or tune the parameters to change the behavior of the tool, so as to avoid detection. It’s usually a trial and error process because in most cases, the designer does not know how the defense system was able to trace him (the artificial user).
Artificial users and main pain points
Every Internet-connected business wants to believe that users or customers who conduct business transactions on their site are real and not artificial users who emulate human behavior and thus, misuse the service in a way that may result in pure revenue loss. Historically, Internet-connected organizations have suffered from a phenomena called spoofed Internet addresses,1 which imposed different types of threats such as denial of service and information theft attacks. A major part of today’s and tomorrow’s cyberattacks are generated by completely real source IP addresses and applications – it is the users behind them that are not real. There are different names assigned to these advanced artificial user tools including Bot, Robots, Poker Robots,2 Crawlers, Scrappers, and perhaps a few others.
These tools have a wide impact:
- Advanced application layer denial of service (DoS) attacks which have been seen more and more of over the past two to three years. These attacks misuse the application resource in such a way that results in an increased latency for the user or worse, a complete denial of service condition. In both cases, the user that experiences this will probably prefer to work with another site.
- Competitive intelligence, which enables the competition to dynamically change their price to always be slightly more competitive.
- Playing with odds on gambling sites through “robotic gambling,” thus increasing the probability of winning in an unfair way.
- Information theft, SPAM activities, and more.
All of the above have immediate negative effects on business revenue.
The main issue businesses are confronted with is how to effectively differentiate between a real user who is trying to perform a legitimate business transaction or search and an artificial one. Failing to differentiate accurately one from the other will lead to blocking “innocent” transactions. Only an accurate, timely identification will protect businesses from the threats posed by artificial users.
Affected businesses include online travel agencies, online auction sites, gambling sites, online stores, bank portals, advertising sites, and more. There are different attack methods conducted by the artificial user using tools that cause the aforementioned negative business effects.
These methods aim to compromise legitimate transactions that comply with protocol and application rules. It is usually the sequence, rate, and timing of transactions that makes the behavior a malicious one rather than “innocent.” Below are a few specific attack methods which explain how it works.
Attack Cases
Scrappers
One specific example of artificial user is the scrapper, usually used to retrieve specific business information across different sections on a site or across different sites. This operation may be conducted by one source or may come from distributed sources which are all connected to a central location that analyses the collection of data.
Referring to travel agencies, scrappers will focus on searching for price details and trip packages. Analyzing this data enables them to dynamically change their price and package types so that they are slightly more competitive in order to win the most deals. Again, the problem of differentiating between scrappers that try to gain information through automatic tools and humans that are conducting a legitimate search is not trivial.
In the online gambling industry the situation is even more advanced. For example, if someone wants to play with the odds of a poker game, he coordinates a group of artificial users that can share information between themselves, thus increasing the chances of this group of users to win. Some of these computerized users will also calculate the odds of seeing a certain card, the styles of other players at the table, and more.
Taking into account that these players are artificial (in the gambling industry they are usually called Robots), the perpetrator does not really need to share his “success” (money) with them. Some statistics claim that one in 8 to 15 players at online poker tables is not human, which gives an indication of the reach of this phenomenon.
Botnet
Another example of artificial user is the botnet, which is formed by an army of bots. Each bot can generate different types of application-level DoS attacks.3 They are controlled by a system that syncs an attack to create maximum impact on the target site, thus putting it in a DoS condition. This type of attack is usually done for extortion purposes or as punishment after an unsuccessful extortion attempt by a cybercriminal organization. In this case, the bot will try to emulate legitimate application-level transactions, thus making it more difficult to detect or differentiate between a real user transaction and an artificial one.
There are many other examples of artificial user tools. Some are basic and some are more advanced at emulating human behavior. What is important to understand about these tools is that they usually aim to attack or misuse public sites owned by businesses that want to maintain the privacy of their customers and allow them to make transactions with minimal steps. This, together with the fact that customers themselves would like to remain anonymous, makes the challenge of authenticating users through traditional authentication schemes a very tough one.
Attack life cycle
As mentioned previously, a typical attack life cycle includes an escalation process in which an unsuccessful attack will be followed by an attack that is more advanced than the previous one. For example, an unsuccessful attempt to conduct some level of fraud against a gambling site can result in the escalation of the attack – to become a powerful applicationlevel DDoS attack that will take down the entire site, for all users, for hours or even days.
When analyzing which technologies would best address the aforementioned challenges, it becomes clear that an advanced ecosystem which incorporates a few solutions with different technological approaches should be developed as an optimal defense. The following section describes the technical challenges and applicable technologies.
Solutions
The challenges mentioned are far from being trivial. Tools that try to emulate human behavior are becoming more advanced, to the point at which they support their own learning mechanism to learn real human behavior styles and emulate them (depending upon the type of activity, whether it be searching methods for intelligence gathering or betting activities in the case of online gambling, etc).
The person who controls these tools decides on the level of aggressiveness as a factor of the level of defense being challenged – making the difference between real human user activity and artificial user activity difficult to ascertain.
Having said this, technology cannot completely emulate human behavior as yet, which means that the businesses targeted, at least in theory, can address some of the challenges imposed by these advanced tools through existing and new technologies that will need to be developed. The following are a few technological approaches which have been used for different purposes and can be beneficial in the war against artificial users:
Analytical systems
There are different types of analytical methods that aim to collect valuable information about the activities that users perform on websites and from which conclusions about suspicious behavior can be drawn.
There are online and offline analytical processing engines, all of which are part of a broader category called business intelligence. These analytical systems4 and methods can be very useful in collecting and arranging information in the right context of business logic. By integrating a behavioral analysis expert system5 on top of these systems, powerful information can be retrieved regarding the assessment of user behavior.
To be more practical, let us take the example of a scrapper tool that try to gain competitive intelligence from a travel agency or from an airline website and then explain how the analytical system helps in identifying it. There are two main methods that scrappers use to search a site, vertical and horizontal:
- Vertical search – this method instructs the tool to try and retrieve all the information from the site. It is almost like copying the entire site into another place. Price information is then extracted and arranged in a convenient way for viewing.
- Horizontal search – This search goes directly into links in the website that include required price, e.g., search for the price of all flights from point A to point B for the week.
As you may assume, there is no real reason for these scrappers to hit embedded links that will activate some flash or Java- Script application – as usually no benefit will be gained from this – but we will return to that in a minute when describing an expert rule system.
Analytical systems can collect and arrange website logs in a way that allows for better analysis of what is happening on the site. Then through statistical modeling, a system can automatically identify non-probable transitions a user is making in the website, between one page and another. This analysis can usually be done through a Markov chain process6 that calculates the conditional probability or, in other words, calculates the probability that a human user will transit from one state to another.
Horizontal and vertical searches will usually be detected using this method in near real-time. This identification mechanism can then be further tuned to integrate expert rules.These rules will include different logical conditions comprised of multi-dimensional parameters such as timedomain, frequency-domain, content-based rules, and others.
Before providing an example of an expert rule, let us first define the main difference in behavior between a scrapper and a human user.
Human or scrapper?
A typical legitimate user who visits a travel site would access the home page (or one of the main pages) and then download all available images, JavaScript objects, and texts. He would then continue and drill down into specific travel sections in the site, based on his specific interest in a certain subject until he retrieves all of the required information. The human user will usually spend some time on each page in order to read and decide which link he is going to hit next.
A scrapper that conducts a horizontal search will usually go directly to a specific section in the site without visiting pages that a human user normally would. It will do this across different sections that provide the same level of information it is trying to retrieve for competitive reasons (such as prices). The scrapper user will usually do this faster than a typical human user would as it will not spend time reading each page like a human user and will not download and process java objects and images that are not needed for the purpose of its search.
A typical expert rule that can identify a horizontal price competitive search will look like the following:
“IF the probability violation level is HIGH”(High weight rule)
Description: this rule aims to discover if the user transitions from one page into another are not probable, based on the aforementioned transition probability model (Markov chain process).
AND,
“IF the number of java object downloads is LOW” (Low weight rule)
Description: this rule aims to discover if the user does not download most of the available JavaScript objects in the pages he has visited.
AND,
“If the hit rate level is HIGH”
Description: this rule is designed to measure if the actions that the user performs look suspiciously fast (i.e., no delays between the transition from one page into another).
THEN, “Suspicious behavior level is HIGH”
In the case that all or some of the above rules are not met, meaning that probability of violation is low and the hit-rate is reasonable, then the final decision output will be LOW (i.e., suspicious behavior level is LOW)
The main responsibility of the analytical system is not only to arrange the information so that the expert system will be able to analyze the logs, but also to be strongly connected with the business logic of the site. For example, in the case of travel sites, pages that include prices should be assigned greater importance/ relevancy in the decision making process, i.e., the rule of probability violation around the price pages will get a higher weight in the decision process. The analytical system should contain this information so the decision will be accurate enough.
The above rule is only one example. Other types of expert rules can help to identify fraud activities against on-line gambling sites and other activities. There is no bullet-proof method to overcome the artificial user phenomena completely. However, if used correctly, some applicable technologies can bring the threat down to a satisfactory level.
Mitigation of the artificial user threat
Before thinking about effective actions that can be taken against identified suspicious users, it should be noted that interference with the user experience on a website, which may be the result of an action, is very delicate and should be treated as such. Online businesses such as auction sites, gambling sites, and travel agencies will not allow for the integration of an “authentication” mechanism, nor will they allow for too many “CAPTCHA”8 challenge/response tests to interfere with user activities.
Beyond the fact that these authentication methods are not always technically possible, the main reason that online businesses try to avoid using them is the delay they force on users, thus reducing the probability that these users will return to do business on their site. Statistically, users will simply go to another competing site that does not “disturb” them with too many tests.
The way to solve these mitigation challenges is to use the “action escalation approach.” This approach aims to minimize the impact on the human user experience while presenting a more accurate and adaptive response to the artificial users.
The main idea behind this escalation approach is to first detect suspicious users or source IP addresses based on the analytical systems described in the previous section, and then start and activate a set of actions beginning with the most gentle one that will have negligible impact on the human user, if at all.
Based on a closed-feedback loop the system will decide if an escalation into a stronger action is required or not.
The following is a more specific example for this mechanism:
- The analytical server identifies a source of suspicious activities.
- The mitigation engine only intercepts the sessions which originate at the suspicious source and replies back with a “weak” challenge option: - A weak challenge can be considered an HTTP “meta refresh” response or a redirect HTTP command that forces real browsers to re-initiate their requests automatically. Simple artificial user tools will fail to respond correctly.
- If the suspicious source responds correctly but continues to generate suspicious activities, it means a more advanced tool is behind the operation (if not, then the suspicious flags that were raised were probably false alarms and the human user will be able to continue his activity on the site).
- The mitigation engine raises the level of the challenge to include some customized JavaScript that forces the suspicious user to download and process the object (different types of JavaScript objects can be customized depending on the type of users and protected sites). Most of the advanced artificial user tools will fail to respond correctly, and will be blocked.
- In case the user responds correctly and suspicious activities are still identified, then the system can either generate a CAPTCHA challenge/response test or simply block the source using a simple firewall rule.
The main benefit of this process is that it allows an accurate mitigation process with minimal impact on the user experience. It also presents an adaptive response aimed at dealing with the dynamic nature of behavior types that the artificial user may choose to use. Lastly, most actions described above do not require the human user to go through any test that will interrupt his activities.
The above actions were given as an example only. Other mitigation options may be used, but the main idea is to follow the closed-feedback loop mechanism, ensuring minimal impact on the user experience, while maintaining a high level of effectiveness in mitigating the artificial user’s activities. Actions such as those described above can be integrated directly into the website application or can be implemented remotely on dedicated network devices.
The ingredients already exist in today’s market. They need only to be used in sync to achieve the right solution.
Summary
Today’s Internet lines and wireless networks are the carriers of electronic business events all over the world. Every Internet connected business wants to believe that users or customers that carry out business transactions on its site are real and not artificial users that emulate human behavior and thus, misuse the service in a way that may result in pure revenue loss.
The market is increasingly expecting information security products and analytical systems to seamlessly authenticate a user to make sure that business transactions are initiated by a real user and not by an artificial one. Taking into account that many businesses want to maintain customer privacy, and the fact that customers themselves would like to stay anonymous, makes the challenge of authenticating users a very tough one.
The article described a few methods and systems that can be used for fighting the artificial user phenomena. It is worth emphasizing that the battle is very tough and there is no one complete solution. The good news is that human behavior cannot be completely emulated – something will always be little bit different. This is the key to identifying and overcoming artificial user threats.