Over half of all internet traffic is generated by bots — some legitimate, some malicious. Competitors and adversaries alike deploy “bad” bots that leverage different methods to achieve nefarious objectives. This includes account takeover, scraping data, denying available inventory and launching denial-of-service attacks with the intent of stealing data or causing service disruptions.
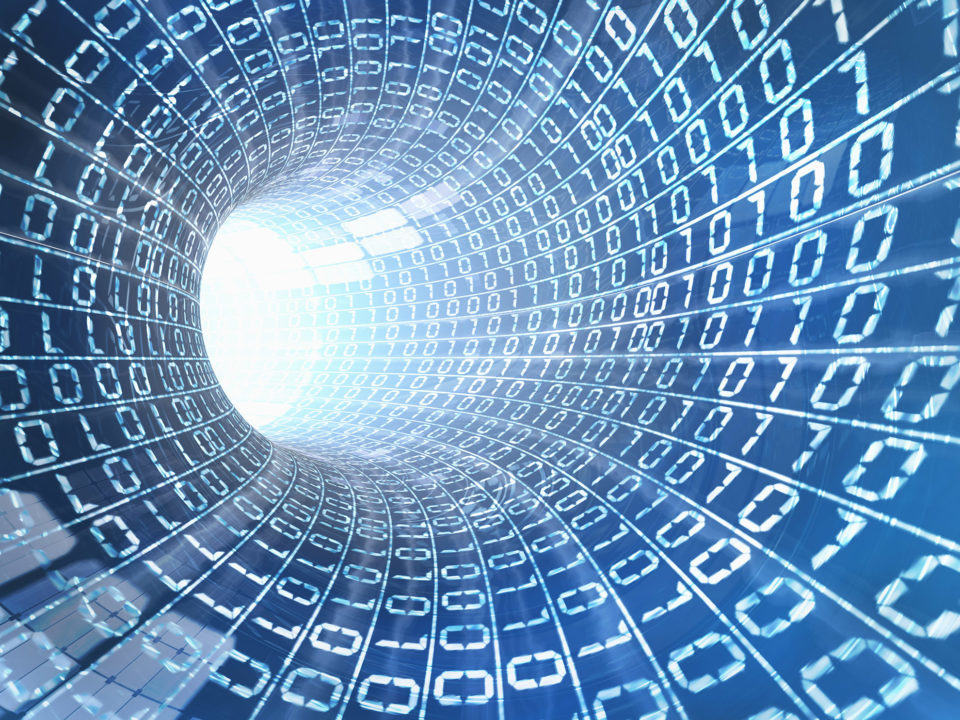
These attacks often go undetected by conventional mitigation systems and strategies because bots have evolved from basic scripts to large-scale distributed bots with human-like interaction capabilities to evade detection mechanisms. To stay ahead of the threat landscape requires more sophisticated, advanced capabilities to accurately detect and mitigate these threats. One of the key technical capabilities required to stop today’s most advanced bots is intent-based deep behavioral analysis (IDBA).
What Exactly is IDBA?
IDBA is a major step forward in bot detection technology because it performs behavioral analysis at a higher level of abstraction of intent, unlike the commonly used, shallow interaction-based behavioral analysis. For example, account takeover is an example of an intent, while “mouse pointer moving in a straight line” is an example of an interaction.
[You may also like: 5 Things to Consider When Choosing a Bot Management Solution]
Capturing intent enables IDBA to provide significantly higher levels of accuracy to detect advanced bots. IDBA is designed to leverage the latest developments in deep learning.
More specifically, IDBA uses semi-supervised learning models to overcome the challenges of inaccurately labeled data, bot mutation and the anomalous behavior of human users. And it leverages intent encoding, intent analysis and adaptive-learning techniques to accurately detect large-scale distributed bots with sophisticated human-like interaction capabilities.
[You may also like: Bot Managers Are a Cash-Back Program For Your Company]
3 Stages of IDBA
A visitor’s journey through a web property needs to be analyzed in addition to the interaction-level characteristics, such as mouse movements. Using richer behavioral information, an incoming visitor can be classified as a human or bot in three stages:
- Intent encoding: The visitor’s journey through a web property is captured through signals such as mouse or keystroke interactions, URL and referrer traversals, and time stamps. These signals are encoded using a proprietary, deep neural network architecture into an intent encoding-based, fixed-length representation. The encoding network jointly achieves two objectives: to be able to represent the anomalous characteristics of completely new categories of bots and to provide greater weight to behavioral characteristics that differ between humans and bots.ли>
[You may also like: Bot or Not? Distinguishing Between the Good, the Bad & the Ugly]
- Intent analysis: Here, the intent encoding of the user is analyzed using multiple machine learning modules in parallel. A combination of supervised and unsupervised learning-based modules are used to detect both known and unknown patterns.
- Adaptive learning: The adaptive-learning module collects the predictions made by the different models and takes actions on bots based on these predictions. In many cases, the action involves presenting a challenge to the visitor like a CAPTCHA or an SMS OTP that provides a feedback mechanism (i.e., CAPTCHA solved). This feedback is incorporated to improvise the decision-making process. Decisions can be broadly categorized into two types of tasks.
- Determining thresholds: The thresholds to be chosen for anomaly scores and classification probabilities are determined through adaptive threshold control techniques.
- Identifying bot clusters: Selective incremental blacklisting is performed on suspicious clusters. The suspicion scores associated with the clusters (obtained from the collusion detector module) are used to set prior bias. ли>
ли>
[You may also like: The Big, Bad Bot Problem]
IDBA or Bust!
Current bot detection and classification methodologies are ineffective in countering the threats posed by rapidly evolving and mutating sophisticated bots.
Bot detection techniques that use interaction-based behavioral analysis can identify Level 3 bots but fail to detect the advanced Level 4 bots that have human-like interaction capabilities. The unavailability of correctly labeled data for Level 4 bots, bot mutations and the anomalous behavior of human visitors from disparate industry domains require the development of semi-supervised models that work at a higher level of abstraction of intent, unlike only interaction-based behavioral analysis.
IDBA leverages a combination of intent encoding, intent analysis and adaptive-learning techniques to identify the intent behind attacks perpetrated by massively distributed human-like bots.